Artificial intelligence (AI) holds immense potential for transforming business operations, yet many companies struggle to fully embrace it. This reluctance stems from a significant trust gap between AI technology and its users. Understanding the nature of AI, its applications and the reasons behind this trust gap is essential for fostering greater adoption.
Generative AI Is Different from Other AI
Generative AI represents a subset of artificial intelligence focused on creating new content, such as text, images and music, based on the data it has been trained on. Unlike traditional AI, which typically performs specific, predefined tasks like classification or prediction, generative AI can produce novel outputs, often resembling human creativity.
Traditional AI models, such as machine learning algorithms, excel at recognizing patterns and making decisions based on data. They can identify fraudulent transactions, recommend products or optimize supply chains. Generative AI, on the other hand, leverages neural networks to generate new data that mimics the properties of the training data. This capability enables applications like chatbots that can converse naturally, AI art generation and automated content creation.
How AI Is Used in Business
AI offers numerous applications that can enhance business efficiency, decision-making and customer engagement. Some notable uses include the following:
- Customer Service: AI-powered chatbots and virtual assistants provide 24/7 support, answering common queries and resolving issues promptly.
- Predictive Analytics: Machine learning models analyze historical data to forecast future trends, helping businesses make informed decisions.
- Personalization: AI algorithms tailor marketing messages, product recommendations and content to individual customer preferences to increase engagement and conversion rates.
- Process Automation: Robotic process automation (RPA) uses AI to automate repetitive tasks, such as data entry, allowing companies to free up employees for more strategic work.
- Supply Chain Optimization: AI optimizes inventory management, demand forecasting and logistics, thus reducing costs and improving efficiency.
- Fraud Detection: Advanced AI systems detect and prevent fraudulent activities by identifying unusual patterns and behaviors in real-time.
- Talent Acquisition: AI streamlines recruitment processes by screening resumes, conducting initial interviews and matching candidates to job profiles.
Why Do We Struggle to Trust AI?
Several factors contribute to the trust gap between AI and its potential users.
For starters, lack of transparency can be a problem because AI systems, especially those based on deep learning, often operate as “black boxes,” making it difficult for users to understand how decisions are made. Concerns about how personal data is collected, stored and used by AI systems also can deter companies and consumers from fully embracing the technology.
Also hindering trust in AI are instances of AI failures or inaccuracies. These events quickly erode trust because users must question the reliability of AI-driven decisions. AI models also can inadvertently perpetuate biases present in training data, which can lead to unfair or discriminatory outcomes.
Another issue that raises red flags for companies and consumers involves ethical considerations. The ethical implications of AI, such as job displacement and the potential misuse of AI technology, raise concerns among stakeholders and foster resistance to AI.
Finally, because generative AI is just taking off, governments lack clear regulatory frameworks governing AI use, adding to the uncertainty and apprehension about adopting the technology. At present, numerous countries throughout the world, including the United States, European Union, China, Brazil, Japan and Israel, are in the process of creating, enacting and enforcing a variety of AI laws and regulations.
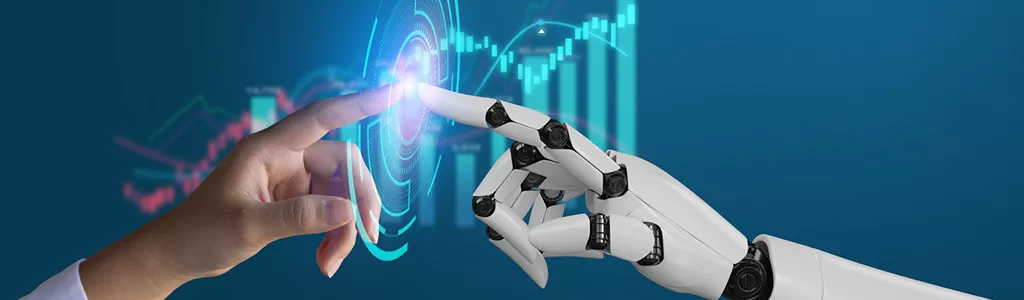
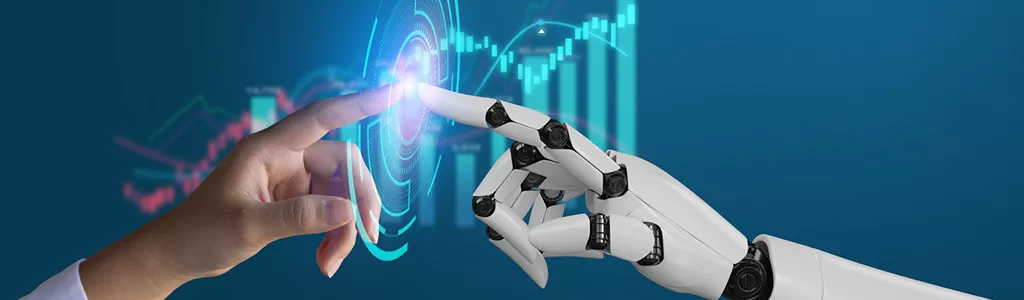
What Companies and Consumers Need To Trust AI
Building trust in AI requires concerted efforts across multiple dimensions
- Transparency: Developing explainable AI models that provide insights into how decisions are made can enhance understanding and trust.
- Bias Mitigation: Implementing rigorous testing and validation processes to identify and mitigate biases in AI systems is crucial for ensuring fairness.
- Data Governance: Establishing robust data governance practices that prioritize data privacy and security can alleviate concerns about data misuse.
- Reliability Assurance: Continuously monitoring and improving AI systems to ensure high accuracy and reliability builds confidence in their outcomes.
- Ethical Frameworks: Adopting ethical guidelines and principles for AI development and deployment helps address broader societal concerns.
- Regulatory Compliance: Complying with emerging regulations and standards can provide a sense of security and legitimacy to AI applications.
Bottom Line
The AI trust gap presents a significant barrier to the widespread adoption of artificial intelligence in business operations. By understanding the nuances of AI, its applications and the underlying reasons for mistrust, governments and companies can take proactive steps to bridge this gap. As efforts to bridge the AI trust gap progress, businesses can unlock the full potential of AI, driving innovation and growth in a trustworthy manner.
About Gravital
Gravital is a performance- and growth-driven digital marketing agency and consulting firm specializing in marketing solutions, growth strategies, measurable results and automation technologies.
Through customized strategies precisely curated by a team of experts in a variety of fields and areas, we close the loop between sales and marketing, make it possible for clients to achieve measurable results, deliver superior ROI, and help companies grow or scale their operations effectively.
We hope the insights you found in this post will inform and inspire your business strategies. If you’re ready to accelerate your growth, we can help. For more information on our services or to schedule a consultation, talk to us today. Let’s grow together!